How do you know if a real estate property is worth the price tag? Or what features are taken into account when valuing a house? Or what pricing model is used to make the estimates or time-value forecasts? These are the challenges of real estate pricing in Data Science.
The economic concept of supply and demand may be instrumental in estimating the price of the house, but it’s not the only factor. Reality is rather multi-dimensional than trivial.
Real estate pricing depends a lot on a huge number of features of the house and maybe its surroundings. And this may also depend on the different buyers’ preferences, adding some subjectivity to the valuation.
It’s indeed as tough as it looks and the work of Danske Bank‘s lead data scientist, Vygantas Butkus, in Big Data-driven real estate price model development proves it.
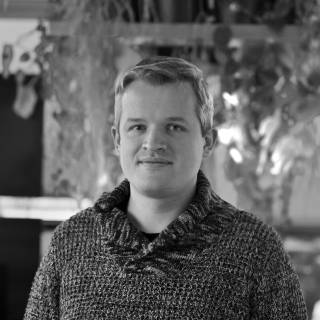
Image Source: Big Data Europe Conference
Vygantas walked the attendees at the Big Data Europe Conference 2019 through a property pricing model he and his team developed in “The Journey Through Real Estate Price Model Development” presentation. You can watch it to learn more.
We approached Vygantas with some questions and found out that employing Big Data in real estate pricing really isn’t a straightforward task.
[Iunera] In your feature engineering demo, you mentioned useless features. Which useless features did you not expect to be useless?
[Vygantas] It is easy to be smart after the research: then you think twice, all features that were not important, were not supposed to be important.For example, the “area of the basement”. The basement itself is important, but the area of the basement is not. Have you ever heard the salesman saying “you know this property is very special? It has extra 3 metre-squared in the basement”? I guess this will not catch much attention.
Another example is orientation of the apartment. This might be important, but it is not. It seems that way more important is what you can see from your window, but not if the window is oriented to the South.
[Iunera] You showed so many models with increasing complexity in your talk. How would a real estate agency know which model is best for the properties they sell? What is the number one criteria in choosing the right model of price valuation for a particular real estate agency?
[Vygantas] Well, the “particular real estate agency” should give some attention to define what is important to them.In addition they should spend some money for Data Scientists who can quantify what was defined.
For example, in our case we were planning to use property predictions to increase User Experience of our product. So, in that case, it is very important not to create bad User Experience.
So, we created an extra model that gives a quality score of the prediction. At the end of the day, we evaluate that percentage of property prices that can be predicted in a defined range of accuracy.
[Iunera] Do the models differ for different real estate agencies?
[Vygantas] No, we were creating a single model for industry, regardless of agencies.[Iunera] Do you think that the level of influence of the features on pricing differ from country to country?
[Vygantas] Not even from country to country, but also within the country. For example, if you take an expensive and dense region in contrast to countryside or a small town. In those two regions you can expect very different behavior.I think comparing country to country is even more complicated. For example, in some countries it is very desirable to own the property.
In a typical scenario, the buyer expects to live in the property – and they take everything very personally.
Meanwhile, in other countries a huge proportion of the properties are rented. Thus, a typical buyer considers this as an investment – “nothing personal, just business”.
[Iunera] Can these models also be used in determining the rental rates for tenants who need to pay rent to the landlords who own the properties the tenants live in?
[Vygantas] I think yes. We know that property prices and rental prices follow each other very much.Thus, if you can predict property price, you can scale it to rental rates. Obviously more research needs to be made in order to make proper scaling.
[Iunera] Has there ever been any case of racial/social discrimination in pricing properties when creating a property contract for a particular racial/social group? Like, increasing the price for a minority group or giving discounts to the dominant group? Can Big Data tackle discrimination issues?
[Vygantas] I am sorry, but we have not considered this scenario. Based on our experience, this would be a very challenging task.[Iunera] Here’s a hypothetical situation. Imagine an apartment neighbourhood located in a secluded sub-urban area, surrounded by green hills and plenty of trees. The apartment blocks are built around a man-made lake and a jogging track circling the lake. So, we have apartment units facing the lake and units facing the outdoor parking spaces next to the apartment boundary fence, beyond which are the forested hills. In your opinion, assuming that other features are the same, which units would be more expensive? Facing the lake or facing the outdoor parking space plus hills?
[Vygantas] I have no idea and I don’t want to make a guess, because I don’t have any solid arguments.There are different buyers who appreciate different views. For example, young happy couples might prefer to see nothing but hills. But once they have children and those children want to go out by themselves, the situation changes.
The same not so young couple now prefers to see a jogging track circling the lake. The prices depend on demand. I have no idea which kind of apartments have a bigger demand.
[Iunera] Now, let’s look at another hypothetical situation. Imagine a neighbourhood of villas in a rural area with a beach and a forest on opposite sides of the street. A row of villas lie next to the beach while the other row is nestled against the forest. In your opinion, again assuming that other features are the same, which row is likely to be more expensive? The beachside villas or the forest villas?
[Vygantas] Sorry, this situation is too theoretical for me.What I have learned, model can predict regular houses. Once the properties become “special” it goes out of the scale.
Writer’s note: This interview has opened my eyes to how real estate-pricing and price model development cannot be taken lightly.
Perhaps, certain factors have a standard influence on the value of a property. But, only to a certain extent.
As more features are added as pricing variables and these features tend to be subject to various opinions, valuation gets more complicated.
I think that the last sentence Vygantas said to me, about estimating property prices based on special features, pretty much says it all.
Since I find it worthy of keeping in mind when it comes to this complex topic of putting a price tag on a property, particularly with Big Data, allow me to quote it again:
“… model can predict regular houses. Once the properties become “special” it goes out of the scale.”
Vygantas Butkus, 2020.