Most of us would know by now that the Covid-19 pandemic has sent shock waves of disruptions to travel plans worldwide as restrictions on travel have been implemented and flights were cancelled.
This has resulted in tourists rushing to cancel their hotel and tour bookings. In fact, the global travel industry has been overwhelmed at the large number of cancellations spurred by the coronavirus.
However, hotel cancellations are nothing new. I’ve come across articles (like this, this and this) that touch on hotel cancellations way before China added “Covid-19” to their vocab.
It’s just that common, so let’s take a look at this issue of booking cancellations that annoy hotels so much that they’re tempted to be less hospitable.
At the same time, we decided to contact one person with familiarity of the forecasting process for hotel booking cancellations, Data Scientist and Time-Series Specialist Michael Grogan, to weigh in on the topic. Our interview is at the end of this article.
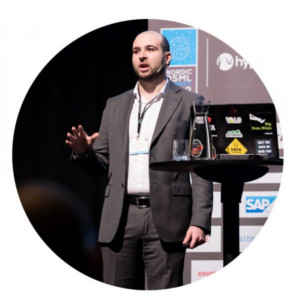
Image Source: Michael Grogan’s website
What is up with people and their tendency to cancel their scheduled hotel stays?
It seems that online bookings, particularly via online travel agencies (OTAs) like Booking.com, Expedia, Traveloka, and Agoda, have made it so much easier and cheaper for anyone to book a hotel room and cancel when the need arises (and even rebook if they find a cheaper deal for the same room). They all do that by using plenty of Data Science techniques.
Numerical data in 2016 showed that most cancellations are made through OTAs, with Booking.com taking the top spot at 57% and Expedia at 26%, in comparison to the official hotel websites with an average cancellation rate of 14%.
It looks like going old-school with the offline or direct hotel bookings is more likely to motivate a customer to not cancel the booking, maybe due to the higher level of effort, time and money used for the direct booking.
But technological advancements is not the only reason hotels see more cancellations. It turns out that psychology plays a role in this as well.
Consumers are always looking for ways to minimise their cost of buying something, so if they found out that they can buy the same thing at a lower price than they paid for, they would attempt to cancel and repurchase, and that’s what usually happens with hotel bookings.

Image Source: Where Can I Fly
But we can’t pin all the blame on OTAs. They’re just there to facilitate bookings in the era of convenience. Hotels may be equally responsible as they’re the ones setting the rules regarding bookings and cancellations. Yet they can allegedly get pretty complacent about how they handle the problem.
Another possible factor of cancellations is the lead days, i.e. the number of days between booking and check-in. Bookings with more lead days have a higher likelihood of being cancelled.
My guess is that having more time until the scheduled stay would give the customer more time to change their mind about the booking or that something else would have happened within that period of lead days that would disrupt travel plans. Or maybe they’ll just forget about the booking.
But that can easily be tackled if the hotel customer service keeps in touch with the customer in advance of the scheduled stay.
It’s been noted that, if a hotel staff contacts the customer, the cancellation probability reduces by 30%, and if the customer responds, the probability reduces further to 1/3 of the usual cancellation rate. So the lack of engagement could be why people are more likely to cancel.
Interestingly, more customers belonging to some nationalities have higher cancellation rates than customers of other national origins. However, it’s important to note that the rankings of cancellation rates by nationality are split into 2 buckets.
One is that of countries contributing to the largest number of cancellations and the other one is that of countries with the smallest number of bookings but still a high percentage of cancellations (a high proportion of cancellations out of a small number of bookings).
Cancellations really suck
Cancellations can have a bad effect on the hotels involved. A loss of income occurs as a result of unsold rooms and no-shows. A no-show is a cancellation without notice. It’s like getting stood up by your date who doesn’t give a rain check, except that the tragedy from this is not just your hurt feelings but also your lost sales income.
Revenue per available room (RevPAR) is lower when the revenue management is done wrongly and when a cancelled room is sold cheaper at the last minute.
The number of extra rooms that could have been sold is influenced by how well the prices are set in response to the number of bookings and cancellations. However, it’s very difficult to correctly estimate the optimal price, so the hotel ends up selling less vacant rooms than they could have.
And when a hotel is faced with a last minute cancellation and then a last-minute check-in, the hotel can’t do much but sell the room at a much lower price, so there goes the opportunity to earn a higher RevPAR. Damn!
And then, there is also the cost of using the OTA’s services for last minute check-ins. Hotels have to pay a certain fee to an OTA for the OTA to act as their middleman, thus lowering profits.
But if the hotel doesn’t rely on an OTA or doesn’t have an automatic download of bookings and cancellations, then the booking and cancellation time is high, and more time means higher cost.
Using prediction analytics to forecast cancellations
If any hotelier has wished for a crystal ball to foresee if any tourist is going to cancel their booking, fret not. Big Data and Predictive Analytics experts have beaten psychics to this by coming up with prediction models to forecast hotel booking cancellations.
We noticed that there’s no one-size-fits-all approach to this as we came across more than one prediction model.
In a study about cancellations at Portuguese hotels, researchers at Universidade do Algarve used the CRoss-Industry Standard Process for Data Mining (CRISP-DM) to make the forecast.
Meanwhile, our interviewee Michael has discussed using TensorFlow Estimators and Long-Short Term Memory (LSTM) to forecast average daily rates (ADRs).
Minimising the impact of cancellations
We’ve explained why cancellations happen and why it sucks, so it’s clear that getting the help of Big Data analytics has so much importance in predicting cancellations.
At the same time, fortunately, hotels can take other steps to alleviate the pain of bookings being cancelled.
- Recording measurements of the hotel bookings and cancellations such as the percentage of cancellations per channel, per month, per room type, lead days, et cetera would be helpful in understanding the problem, especially when deploying data analytics.
- Engaging more with the customers who booked stays of higher value prior to their stays to hopefully reduce their likelihood of cancelling the booking.
- Keeping an eye on the pricing of the hotel stays on other channels, including OTAs that the hotels are not partnering with. This is to ensure that their customers don’t jump ship to re-booking their hotel rooms on other channels at lower prices.
- Implementing a strict cancellation policy with conditions like a 24-hour notice, deposits, non-refundable rates and length of stay restrictions to make sure that the matter of bookings and cancellations are taken seriously by the parties involved.
Interview with Michael Grogan
As we mentioned earlier in this article, we reached out to Michael Grogan to share his insights and opinions about hotel booking cancellations and using Big Data techniques to forecast cancellations.
Feel free to check out his talk about the topic in the video.
Without further ado, here’s our interview with him:
Iunera: On average, how much can a hotel lose from each cancellation?
Michael: This is highly dependent on the hotel in question.
However, analysis of a public data set for a Portuguese hotel (data sourced from Antonio, Almeida and Nunes (2016) of Universidade do Algarve) showed that the mean ADR (average daily rates) for customers that cancel (105 per customer) were higher than those who followed through (90 per customer).
Moreover, 27% of overall bookings were ultimately cancelled. Therefore, there is evidence that revenue loss from cancellations can be substantial.
Iunera: How does re-booking impact hotels economically? Can hotels ever gain from a re-booking? And can prediction models take re-booking into account?
Michael: It depends on the reason for the re-booking. e.g. a customer might make a booking with a cancellation option at a higher price. However, if the customer is then certain of their booking later, they may choose to pay a non-refundable option at a cheaper price.
From this perspective, hotels can gain from this type of re-booking as it is good for cash flow (since the payment is made earlier), but the trade-off is that the customer books at a cheaper price.
Prediction models may be able to take re-bookings into account if the individual customer can be identified in some way, e.g. a booking ID. However, this depends on regulations – e.g. it could be the case that due to customer privacy issues – any data used for modelling purposes must be anonymised.
Iunera: What are the pros and cons of LSTM and TensorFlow Estimators?
Michael: LSTMs are advantageous in that they can model volatile time series better than ARIMA in some cases. However, ARIMA is still superior when it comes to modelling data with a defined trend.
TensorFlow Estimators is quite effective when it comes to a “scenario analysis” type of forecast – e.g. modelling numerous forecast paths, which would have been useful pre COVID-19. However, the disadvantage is that such forecasts are less rigid and subject to human interpretation.
Iunera: What are the pros and cons of the CRISP-DM model (used by the Universidade do Algarve researchers in their study) to predict cancellations in Portuguese hotels?
Michael: CRISP-DM can be advantageous in that it uses an AGILE methodology for data mining and analytics. This can minimise wasting time on collecting the wrong data or building the wrong models, for instance.
However, this can be a double-edged sword in that the ultimate outcome of the study remains uncertain and there is no guarantee that the data mining or analytics processes will produce a useful outcome from a business standpoint.
Iunera: How do we know if a prediction model works for a particular hotel but does not for another hotel?
Michael: This is a difficult issue, especially given that data on this topic is not widely available due to privacy issues.
However, analysis of two separate Portuguese hotels based on public data showed that models built using one set of hotel data showed strong predictive power in predicting cancellations for another Portuguese hotel.
However, it could be that cancellation trends for Portugal are different from that of hotels in other countries, and therefore more research is necessary to answer this question.
Iunera: What are the potential challenges/barriers/obstacles to forecasting hotel booking cancellations? What are the challenges of obtaining the data? Which special features should be contained in the data to make predictions work?
Michael: As mentioned, the primary obstacle is a lack of data on this topic – the main challenge being that customer privacy is an important consideration in this industry.
Based on the public data sets from Antonio, Almeida and Nunes (2016), it was found that factors such as lead time, country of origin, and time of year were significant influencing factors.
Iunera: How can hotels/accommodation owners avoid/minimise losses from booking cancellations and re-bookings using the predictions?
Michael: While a hotel can never eliminate this risk, many currently charge higher prices for inclusion of a cancellation option, which helps mitigate the risk somewhat.
Moreover, they might choose to refocus their marketing efforts on customer segments with a lower degree of cancellations.
Iunera: Here’s a tough one: Should decisions based on the cancellation
rates by nationality be made to act upon guests from countries with
high cancellation rates?
Michael: A tough one indeed, as such an issue gives rise to price discrimination or other anti-competitive concerns.
A hotel might use this data to increase their marketing presence in certain countries over others, or they may choose to take a more aggressive approach in terms of different pricing tiers, etc.
However, this issue is regulatory in nature and I wouldn’t be able to comment as to how pricing regulations work across this particular industry.