What are the key technical challenges when starting Big Data-driven decision making and Data Science application development? What are the threats of transforming an organization into a Big Data-driven enterprise? We describe some fundamental organizational and technological challenges and how you can spot them earlier.
What you learn in this article:
- What are some key problems in developing Data Science Apps and becoming a Big data-driven enterprise?
- Which manifestations appear when Data Science projects are getting off-track?
- Which first countermeasures can you introduce when the manifestations appear?
- What can you learn from Highlander in defeating the four horsemen of Big Data Science?
- Outline
- The four horsemen of Data Science Apps
- White Horse / Conquest of Big Data analytics
- Red Horse / Data Science War
- Black Horse / Starvation of Data Science Projects
- The pale Horse / Top-down Death of the Big data-driven enterprise
- An alternative solution by Highlander
- When you defeat the horsemen, people will sing praises about you
- Related Posts
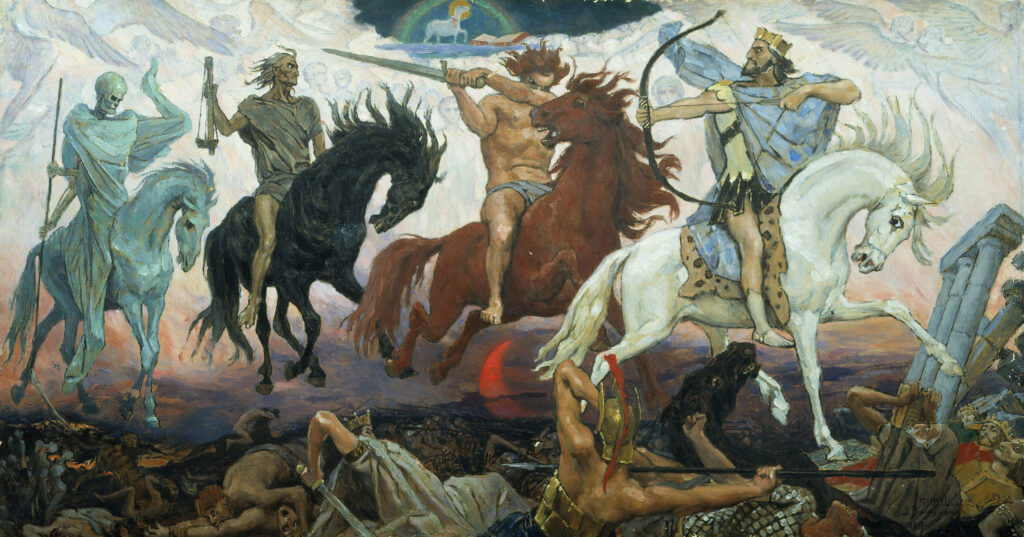
Source: Viktor Vasnetsov
Outline
The four horsemen represent the different levels of problems, whereby there are different levels to how projects can go wrong in a Big Data-driven company. We describe four different problems/horsemen which cause the development of Data Science Projects/ Data Science Apps (DSAs) to suffer and ultimately fail.
For each scenario, we dig into appearing symptoms and resulting implications for the enterprise, when the projects are not “healed” and how they jeopardize top Data Scientist talent motivation and acquisition.
Using examples, we elaborate on how to spot the threat of the specific challenges faster. Then, we point out the first solutions and directions to overcome and defeat the problem.
Finally, we provide conclusions and see why the human element in the age of artificial intelligence is equally important as in each time before.
The four horsemen of Data Science Apps
The four Horsemen of the Apocalypse of John are described in the Bible’s New Testament. They symbolize a stage of the end of the world towards the last judgement. In order to digitize a company successfully and to get sustainable Big Data projects running, there are obstacles that can be as hard to overcome as annihilating a horseman.
One method to unlock value from Big Data Science is to develop intelligent applications processing Big Data and acting on proactive decisions founded on Big Data.
This is what we call Data Science Apps (DSAs). Such applications can be predictions, hyper-personalization, recommendations in an online store, pro-active discounts, cross-selling or up-selling based on user navigation and much more.

In order to develop and deploy such a Data Science App (DSA) in production and leverage company value from it, several struggles and threats have to be tackled. I call them the four horsemen of Big Data-driven enterprise.
- White Horse:
Conquest of Big Data - Red Horse:
Data Science War - Black Horse:
Starvation of Data Science - The Pale Horse:
Top-down Death of the Big Data-driven enterprise
Each one attacks the Big data-driven enterprise in its own way and leads to pain and suffering in digitizing an enterprise. The challenge for you as a reader, should you choose to accept it, is to learn to spot them and to introduce countermeasures.
Let us start with learning how to slay the white horse rider:
White Horse / Conquest of Big Data analytics

Source: Viktor Vasnetsov
Conquest can destroy Big data-driven applications. There can be a false belief about techniques that resemble real Big Data-driven applications and decision making, but are actually not and all they do is mess up the project.
A common example are projects that are set up in a non-scientific manner and are there just for the sake of jumping on the technology bandwagon (“we need to digitize”, “we need to do a project with Data Science, AI, forecasting or Big Data-driven applications”).
There are also digitization projects set up, just to prove a point by cherry-picking data that supports this person’s opinion and ignoring data that proves otherwise, instead of objectively gathering and analyzing data as it is.
Worse comes to worst, the biases are not addressed or the data quality is overlooked. The pressure increases step by step to deliver the project outcome as promised and the rare top talent gets burned out more and more.
Hunt the white horseman with dedication
Normally, you spot this rider by seeing strong tool orientation. Often also project teams “wait for data” from another team.
Classically, such projects run for months without delivering concrete examples of how to act on data and they are defined by planning what needs to be done to get started.
There seems to be a masterplan of what kind of miracles are happening once all the data arrives. Ultimately, there is a paralysis of progress because all wait for the big bang that enables progress.

Source: JACLOU-DL
Slay the horseman with conviction and preach right values
Once you spot this horseman, shift the project into a scientific manner. Clear and concise communication to the (top) management is required that you can only measure assumptions and not allow biases to influence the data.
Ultimately, hypothesis need to be formed and the data access needs to be democratized in order to let investigations of hypothesis compete.
With such democratized Data Science, hypotheses get formed, and then the data investigations are done on a small sample. Subsequently, the investigation outcomes get studied and discussed and new hypotheses are formed. Then, the data sample gets extended, the hypothesis gets verified more deeply and more insights get revealed.
Once the manually investigated results get to a certain stability the next steps are operationalizing a small scenario based on that data. It is not important that it is huge, it is important to return the first value to the stakeholders of the project. Once this is done, one needs to iterate, improve and extend.
Red Horse / Data Science War

Source: Viktor Vasnetsov
The second horseman comes with a sword ready for battle and mass slaughter. It might first seem the right way to form a team to stick together and get the “data team” ready for battle. However, rivalry between teams is the wrong way.
Big Data-driven engineering requires cooperation and this means democratization of technology, democratization of data access and also democratization of Data Science.
Big Data-driven engineering also means together and not one against another. The Big Data team needs the other departments as partners to work together. The negative rivalry only results in doom.

Source: blende12
A common example are Big Data and Data Science projects which are set up without the proper management support, resulting in arguments about data ownership or having to beg for data access.
Often there is also technical rivalry. Imagine that a Big Data or data lake team and a Data Warehouse team are competing.
The Data Warehouse team feels threatened by the new Big Data tools and tries to create results on their own platform or to make the access complicated. It’s true that this rivalry happens in different areas, but it is also absolutely human to be uncertain and afraid of the implications.
Clearly, technological battles are not the worst things in this matter, because often technology still stick with technological and logical reasoning. The worst case of battles are the ones where there is a division culture that ends in a certain hostility.
Prejudices
Even though computer science shapes a huge part of this planet, there are still prejudices for computer scientists.
In extreme forms, some people in the non-technical department might even see the development data team as pure suppliers and not as partners anymore. In rare cases, the data team gets labeled as Nerds, Geeks, “the binaries” or island talented.

Source: unknown
On the other side, the data teams can regard other departments as not knowing and “unenlightened”. It might be that the focus gets away from the use case and the purpose of the projects leans more to applying technology and vastly ignoring the needs of the data stakeholder.
Battlegrounds lead to agony, frustration and a hostile work environment. Key problem is that such attitudes can spread throughout an organization, making it incredibly dangerous for the organization.
As a result, top talent might be so frustrated that they’ll end up leaving or not joining the project, ultimately resulting in unfinished projects.
In short, Yoda, the Jedi master from Star Wars would say: Frustration leads to anger, anger leads to hate, and hate leads to suffering.
Following Yoda, It is essential to apply principles of the light side of the force to ensure transformations of Big Data-driven enterprises work smoothly.

Picture credits: EsaRiutta
Beware of Big Data battlefield
This red horseman comes hidden and is hard to spot. Often sabotage or non-existing valuations are hard to recognize in the beginning. The first signs are recognizable when pressure builds up after the first results do not get out in time.
Then, occasionally some finger-pointing can be seen, indicating stakeholders are not looking in the same direction. Subsequently, there is a “we and they” division where project participants create plans on how to manage the other group better.

Source: Tumisu
Commonly, new tools or hiring more resources for management or data analytics are applied to get the project going.
The main problems are that the right data in the proper quality is not available and the problems related to “agile top-down planning” are ignored.
Classically, the employees now deal with activating the new tools like “Data catalogs”, “AI data cleansing tools”, “A special Ai algorithm“, “data visualization tools”, “lambda services” and many other “prerequisites” that are needed before getting a tiny functionality and return live and in production.
Ordinarily, work plans are then lacking sync and when people of the different departments get asked where the project stands and what it will deliver as results, completely different perceptions get told.
Once this point is reached, the project gets torn apart. All the different distractions and huge goals seem to make a small viable first proof of concept that DSAs are out of reach…
Use all of your heart to win the Data Science War epically
Once you spot this horseman, it is integral to have two things to bring it back on track:
- The commitment of each department’s management to ensure that the project participants are acting as one team and follow the same goals.
- The willingness of the participants to respect, listen to and cooperate with each other to align on an iterative approach to achieve results step by step.
The management has to ensure that these values are really lived by. One can imagine such a mission as virtual agile war rooms where development and the data owners are responsible together to deliver results.
The hard part in overcoming this rider is the ego of the different participants where each has to step back a bit to achieve the common goal. Ultimately, this also bears the chance for everyone to grow as a person.
Black Horse / Starvation of Data Science Projects

Source: Viktor Vasnetsov
The third Horseman stands for famine. It might, at first, not look so familiar or relevant, but in reality, this happens to many planned Big Data-driven applications.
The board of the management of a company decides that they need to catch up with what is hip and then some initiative needs to get started.
Once a small team is hired, first results and demos get generated, use cases get engineered and investors/stakeholders/customers for the next steps are needed.
There, a first try might get executed if things are generally possible, but it gets clear that a setup in production needs more time, more resources and more commitment.
We can sum up the situation as follows: Use cases are engineered, the first proof of work has been done, a few Data Scientists are there. There are interested departments, but no real commitment without a reliable success story is existing.
Without commitment, Data Science cannot grow and without growth there is no commitment. A typical chicken-egg situation of getting Data Science and Big Data starts. Overcoming this problem is essential in order to transform into a Big Data-driven enterprise.

Source: Ruben Alexander
Hence, there is the Big Data team hoping for the growth to start. Time passes by and nobody feeds into this situation. All started initiatives starve step by step because nothing is happening.
The worst thing in this situation is that the people involved lose their drive and enthusiasm. Your high-value assembled and initially intrinsically-motivated top-talent is now demotivated and the question only arises when the first team members are looking out for better opportunities.
Nightmares will tell you once the horseman is there
This horseman is quite easy to spot, but sadly, way too common. You normally see it when you give suggestions to the team members on what to do. Once new ideas are proposed they will tell you reasons why this cannot be done (e.g. “our legal department will not allow this” or “we do not have the mandate for that”).
Alternatively, you can also measure it by progress and a lot of defined use cases where none have been implemented and there are lots of constraints and future plans. Everything seems to be built on a miracle that comes and then all problems are solved.
When one studies the initial constraints and then returns for the progress after a few weeks, there is still no measurable progress. Instead of progress, old use cases have been defined as lesser priority or not doable due to constraints and now new use cases with plans are there.
Fight starvation with bread and games
In order to fight and defeat this horseman, we need to fight the root cause. The key problem of this situation is the lack of resources to accomplish a major task that all people expect from the current hype.
A Russian saying for this situation is:
двумя зайцами погонишься – ни одного не поймаешь
“Chasing two hares – you won’t catch a single one”
In order to overcome the challenges, a focus on a single and minimum viable use case is needed. A focus also means that all eggs are in one basket and if this use case does not deliver the value, a problem occurs.
Additionally, green light reporting that everything works needs to be switched off to be able to address the root causes and constraints.
Therefore, the senior management needs to be involved in backing the project upon failure or helping to overcome the constraints with initial sponsoring. Without this backup, panic or the lack of resources will always lead to fractionation and loss of focus.
A regular and honest (not green light reporting) structure needs to be established where evidence of progress for all stakeholders is provided. This ensures that the project stays on track and in addition, the resources which are in the project get utilized with the necessary credit and find their motivation again.
The pale Horse / Top-down Death of the Big data-driven enterprise

Source: Viktor Vasnetsov
The pale horseman comes with the power of plagues and epidemics.
Big Data-driven projects are naturally not started on green meadows. The projects are based on technological and organizational foundations which are already existing in an enterprise.
When Big data-driven projects are set up in the wrong manner, the problems which already exist in other projects spill over to the planned DSAs.
Another issue is that many organizations are traditionally not software vendors. Hence, becoming a Big Data-driven enterprise is a new approach for the whole organization. Traditionally, such enterprises often work in typical top-down planning ways.

Source: Bright Hub PM
Consequently, adopting “agile” processes often results in “agile-waterfall” methods where planning gets done top-down. Such projects are crippled from the start and never have a real chance to grow.
Planning top-down is a plague that most Big Data Science projects do not survive. There are various reasons why this top-down approach does not work:
Data integration efforts are underestimated
Technologically, efforts of data integration are underestimated topics. After all, at least 80% of the work is data preparation and integration. Furthermore, additional data cleansing might also be needed.
Commonly, the use cases of the Big Data project get evaluated by different people who know the data sources and efforts in integration. Thus, the effort planning is more like a crystal ball prediction than a real expert opinion.
Once the project gets confronted with the reality of data integration effort and data quality assessment for a real productive application, major problems arise.
Tools are expected to solve human problems
Worst comes to worse, teams attempt to solve data integration problems with tools and not by adjusting processes. Tooling for data integration get introduced in hopes that technology can solve expectations and speed up the implementation.
In order to apply the tools quickly, the best practices of the vendor get almost completely ignored. Such ignored best practices are normally to apply the tools in agile processes and to stick to typical software development of methods like testing and versioning.
The irony is that death comes from within and slowly after time, while everything looks fancy. Basically, the described organization has all the tools they need but is crippled by processes which do not align to software development resulting in an inability to manage and control the technology.
Follow the smell of decaying data
This horseman can be detected by looking at how goals are set and how processes are implemented. It is unimportant if the processes are named Scrum, Kanban, or similar, but it is important how they are executed. If there is total top-down planning, you spotted the horseman.
In extreme cases, there might even be someone who says “I have an idea” and proclaim the idea is the most important in a project. However, having ideas and planning top-down is essentially not seeing that Data Science needs to be executed in an iterative cycle.
Embrace Death with friendship, openness and rule as emperor
This horseman is hard to come by, because the setup has been created top-down.
Therefore, the metrics and failings have to be communicated properly to allow the senior management direct insights on why traditional top-down approaches do not work here.
Once there is a commitment it is important to get a first application running and to generate a success story. This works as follows:
The desired outcomes and values of the Big Data-driven enterprise need to be clustered into different scenarios. Once identified, the scenarios then need to be structured for their value and data access.
Now, the application with the easiest access to the data needs to be identified. Then, this application needs to be developed and deployed in an iterative manner.
I personally recommend working together with an external company that gets the mandate to manufacture the first Data Science App outside of the company. This avoids growing interdependencies and to have a proper template for a working Big Data Science App.
Once the first application has been developed successfully this application can be extended. At the same time, the other scenarios can be manufactured in the same manner and the knowledge of the team can be spread.
An alternative solution by Highlander
For the sake of completeness, I mention here another way to defeat the riders in Highlander. The highlander series double episode “Comes or horseman” discusses the topic of the horseman. Likely, you also know what is done there: Their heads are chopped.
At this very moment, I beg you not to apply this practice in your companies.
When you defeat the horsemen, people will sing praises about you

Source: azboomer
We discussed the different challenges to develop Data Science Apps and executing projects to realize them.
The white horse destroys Big Data projects by introducing the wrong beliefs. This one would likely lead to a loss of top talent.
The red rider represents the war between different teams. Battlegrounds lead to agony, frustration and a hostile work environment for Data Scientists.
Starvation in the form of the black horse represents an improper commitment to Data Science, resulting in limited or no growth. Ultimately, a typical chicken-egg situation corners to get Data Science and Big Data-driven enterprise started.
Finally, Death on the horse represents waterfall project management and using technology to try to solve non-technological problems. This might not just jeopardize the Big Data Science app in development, but also imply future cost factors for the Big Data-driven enterprise.
The challenges are mostly founded because the dynamics and beliefs of different stakeholders are not aligned. In fact, most of the problems, even then technological challenges, need a human interaction component to be resolved. Out of this, we conclude:
Regardless of how much technology and Data Science we use, humans are still needed to talk to one another and to look in the same direction. Together, team players in an Big Data-driven enterprise can defeat the four horsemen of Data Science Apps.
Get in touch with us
If you are interested in Fahrbar or want to find out how we can help you leverage your data