Data Scientists often do not consider master data as an important topic. Consideration of master data is often crucial when working with different data sources in the Big Data area. Hence, this article describes what Master Data is, how it’s so important and in which areas it matters most.
What is master data?
Organisations usually deal with a few main types of data, including unstructured data, transactional data, metadata, hierarchical data, reference data and, of course, master data.
Yes, master data is just one type of data inherent in organisations.
A commonly quoted definition of master data is Gartner’s definition.
“Master data is the consistent and uniform set of identifiers and extended attributes that describes the core entities of the enterprise including customers, prospects, citizens, suppliers, sites, hierarchies and chart of accounts.”
Gartner
According to Profisee, master data consists of four domains (which may vary across different organisations):
- Customers: Within the customer’s domain, there are customer, employee and salesperson sub-domains.
- Products: Within products domain, there are product, part, store and asset sub-domains.
- Locations: Within the locations domain, there are office location and geographic division sub-domains.
- Other: Within the other domain, there are things like contract, warranty and license sub-domains.
Whatever the domains of master data are, three characteristics about master data remain the same for every organisation, according to Semarchy:
- Critical for business decision-making.
- Scattered throughout the organisation.
- Represents a “single source of truth”, in other words, the “core data”.
With that said, maybe Dataversity’s definition of master data is the clearer one.
“Master data can be described as an organization’s core data, containing the basic information needed to conduct business.
It is fairly stable information, changing only when something dramatic happens, such as a client moving to a new location.”
Dataversity
How does it differ from time-series data?
To explain how master data is considered as stable, here’s a comparison with time-series data. Time-series data are actions, events and/or indicators which occur at different times.
The primary factor of time-series data is the focus on the time dimension.
This means that the point in time of an event is considered as a new data record, which can be compared to past records as part of a time-series analysis.
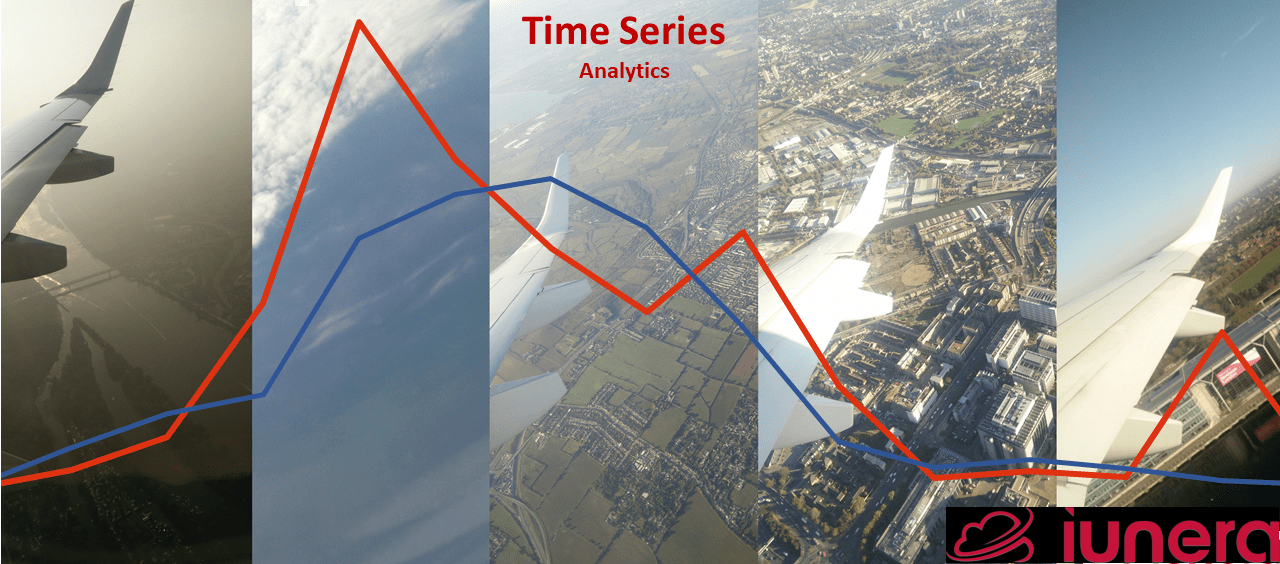
Another crucial factor of time-series data is that its underlying concepts drift over time.
On the other hand, master data doesn’t experience such changes unless, as mentioned earlier, something dramatic happens.
If the master data changes, everything changes and this phenomenon of master data change is referred to as “slowly changing dimensions”.
The changing dimensions of master data has to be credited in the analysis for it to make sense.
In the case of a commuter bus, there are temporal concept drifts on weekdays and weekends.
On a Monday, which marks the start of a work week, many passengers use a particular bus route, causing a delay in the departure from each stop.
On a Saturday, less people use the bus, resulting in less delays.
Since the bus route is always the same and the delays differ from time to time, the bus route is the master data while the daily delay is the time-series data.
On rare occasions, the transport department decides to adjust, expand or shrink the bus route to better accommodate the commuters’ demand in that town.
Data scientists will have to take note of the adjustment in the bus route since it will shift the passenger numbers and delays.
In another example, you use a Facebook account that has all the personal info associated with you, like your relationship status, photos, links, opinions, participation in groups, the pages you follow, etc.
The Facebook account acts as your master data because it’s the core of all the online data associated with you.
Meanwhile, your Facebook activities, which happen at different points in time, are considered your time-series data.
The same can be said in the case of Twitter.
You have the Twitter account acting as master data while the Tweets are time-series data.
Your social media activities (time-series data) may differ with time but your social media accounts (master data) remain as they are, unless you deactivate them.
What is master data management (MDM)?
In their 2007 publication Master Data Management and Customer Data Integration for a Global Enterprise, Alex Berson and Larry Dubov (quoted by Mike2.0) define MDM in the following way:
“Master Data Management (MDM) is a framework of processes and technologies
aimed at creating and maintaining an authoritative, reliable, and sustainable, accurate, and secure data environment
that represents a ‘single version of truth,’
an accepted system of record used both intra- and interenterprise across a diverse set of application systems, lines of business, and user communities.”
Alex Berson and Larry Dubov’s definition of MDM
This basically means that MDM is the idea of using tools and/or setting procedures to maintain the qualities of the organisation’s core data for everyone’s benefit.
Referring to Dataversity’s explanation, MDM aims to achieve the following qualities:
- All of its essential data in one master file for the entire organisation’s common reference.
- Elimination of bad data governance.
- Uniformity and accuracy of data.
- Streamlined data sharing between different users and departments.
So, the basic idea of MDM is consolidating and maintaining this core data to make it easier for the relevant parties of an organisation to access it.
What’s the big deal with MDM?
A business is nothing without its domains of customers, products, employees and legal documents.
We mentioned earlier that master data consists of these domains, hence, it plays a big role in keeping the business alive if managed properly.
And because master data is used by various parts of an organisation for different applications, an error in one part of the master file can cause errors in all the applications that use that part.
Here’s a classic example of how an error in the master data can be detrimental to an organisation’s operations:
An e-commerce business sold a product to a customer. However, the business later harassed the customer with ads of the same product that the customer no longer needed.
Now, you might be thinking how the business could allow this to happen.
The answer is simple: Poor MDM.
In this scenario, the sales team and the marketing team did not integrate the customer’s information.
The marketing team was unaware of the sale, so they ended up wasting everyone’s time.
Therefore, the solution to such an annoyance is for the sales and marketing teams to coordinate by using the same master data.
Okay, so how can master data be created and maintained?
This is why the power of master data and its management cannot be underestimated.
Creating master data involves cleaning and standardising data, and matching data from all the sources to consolidate duplicates.
Examples of data cleaning work are turning all the phone numbers, addresses and other details into common formats, replacing missing values, and converting all measurement units to the industry standard.
Meanwhile, consolidating duplicates requires accurate matches and needs to be done with extra care as false matches can lose data and missed matches defeats the purpose of the process.
It should be noted that there are several MDM approaches and techniques including:
- Migration of historical data
- Application co-existence
- Single copy
- Multiple copies with single maintenance
- Continuous merge
Whatever approach the organisation decides to use, the organisation should be following these dimensions and disciplines of MDM strategy:
- Governance and policy to set MDM rules and standards.
- Measurement for progress tracking.
- Organisation to assign the right team of master data owners, data stewards and governing parties in the strategy.
- Process to determine the steps to manage MDM.
- Quality assurance to uphold data quality.
- Technology, of course, provides the tools needed to make the MDM strategy possible.
The best MDM approach for a particular organisation holds the secret sauce to an organisation’s success.
Therefore, as long as the disciplines above are applied, the master data is in good hands.