Let’s say you’re finding an item in the storeroom. But you can’t find it because of all the other things that are taking up space and blocking your way. Data scientists face a similar issue when finding the data they need amidst the unused data taking up space in the data storage. This unused data is known as dark data. Dark data will be introduced in this article.

What is dark data?
Dark data, also known as dusty data, is the unstructured data that an organisation continuously collects and stores but not organise and use.
“Collected and stored…
NOT organised, NOT used…”
How I would write about dark data in a poetic way.
There are three dimensions of dark data:
- Traditional unstructured data: Traditional unstructured data is text-based data that does not have a uniform structure and is not organised in a predefined manner. It includes the data that is already present in the organisation’s cache such as emails, notes, documents, logs and notifications.
- Non-traditional unstructured data: Non-traditional unstructured data is made up of the hoarded image, audio and video files that cannot be processed or analysed with traditional analytics techniques. More advanced techniques like computer vision, advanced pattern recognition, and video and sound analytics will be needed to process such data.
- Deep web data: Deep web data is said to be the largest body of untapped data curated by academics, government agencies and third-party domains. Examples of deep web data are medical records, fee-based content, membership websites, and confidential corporate web pages.
Organisations usually collect unstructured data “just in case” they need them.
But they don’t know how to use or analyse unstructured data, so they end up leaving the data unused and forgotten.
Just like the items that collect dust in the dark store room at home.
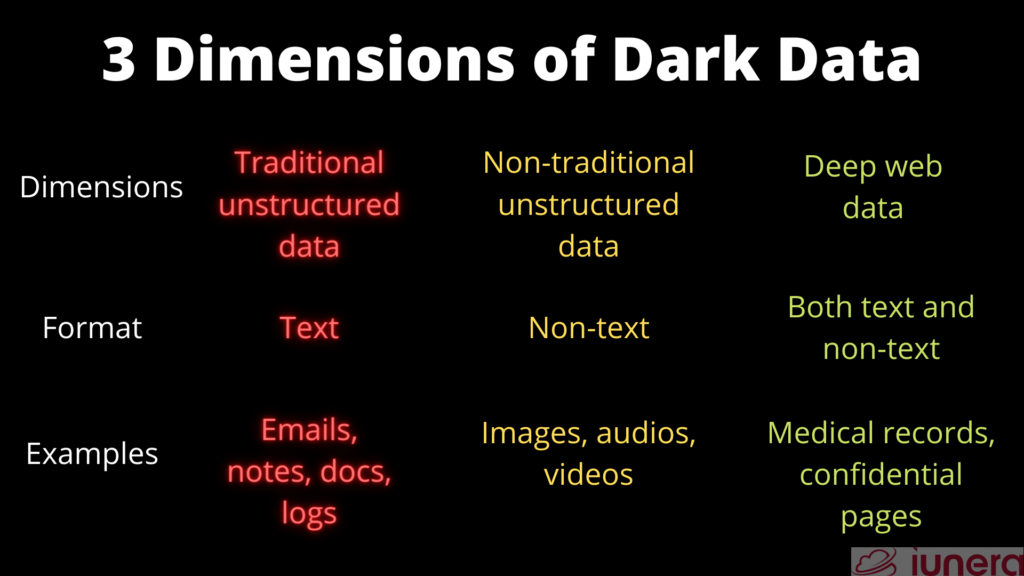
Importance of dealing with dark data
There are several reasons to not leave dark data ignored.
Firstly, dark data affects data quality in terms of accessibility.
Accessing the necessary data is like finding a needle in a haystack consisting mostly of dark data.
IBM estimated that 90% of all data generated is dark data.
So, obviously, this haystack of unused data would be taking up too much storage space, which brings us to the next point.
Storage can be costly, so not making good use of all the data stored including the dark data would be like throwing money out the window.
Another reason to put dark data to good use is cybersecurity.
Most organisations may think that it is useless, so they don’t bother protecting it with cybersecurity tools.
However, hackers might disagree about its uselessness.
Yes, it can actually be valuable if organisations know how to use it, which we’ll get to later.
If hackers can steal the dark data, it might place the organisations at risk.
So, if the organisations leverage it, they’ll have the incentive to protect it from data theft.
Since 77% of managers and executives in the world believe that finding and capturing dark data should be a top priority, let’s look at the ways organisations can do so.
Suggestions for using dark data
According to TechRepublic, the very first step organisations have to take is to find out what they have.
It just makes sense to know what their dark data looks like before they can do anything with it, right?
Once they know what they have, they need to determine if it provides value to their company or not.
If it doesn’t, they can clear the data clutter.
If it does, they can keep the dark data and tap into it.
For the second option, they can add external data to complement the available dark data to support decision-making.
While looking at both dark and external data, they have to check the data’s quality.
Common use cases
At this point, it’s becoming clear that using dark data can uncover important information that help organisations make strategic decisions.
According to Precisely, here are some common use cases:
Customer support logs
A business typically keeps records of customer support interactions in a CRM (customer relationship management) system including:
- when a customer contacted the business
- products or services used
- which communication channel was used
- the pipeline stage
Instead of abandoning such data or using it only when there’s an issue, the data can be leveraged to understand or predict when customers are most likely to make contact, their preferred channels, their preferred products or services, etc.
Non-textual data
Textual data may be easier to process but non-textual data also has the potential to provide insights.
For example, a research publication described how administrative textual and non-textual data from public archives were used to predict the performance of Danish startups for innovation support decisions.
The performance indicators were survival, high employment growths, a return on assets of above 20%, new patent apps and involvement in an innovation subsidy program.
What the research may have considered as non-textual data include the characteristics of the founders and startups that may not be captured in the textual data they obtained.
Legacy system log
There are situations whereby organisations keep older types of systems like a software that works on an older version of the operating system (i.e. Windows).
It would be hard to imagine using modern analytics tools to understand these old systems but it’s actually possible.
These system logs just need to be moved into an analytics platform like Hadoop for legacy system data to be useful.
Lessons
Here’s a little summary of what we learnt from this article:
Dark data is unstructured data collected and stored but not organised and used.
Its 3 dimensions are traditional unstructured data, non-traditional unstructured data and deep web data.
Dark data is normally treated like the dusty, unused items in the storeroom at home because organisations don’t know what to do with traditional unstructured data, non-traditional unstructured data or deep web data.
However, leaving it abandoned can result in issues for data quality, costly storage, cybersecurity risks and missing out on hidden insights.
Dark data can be a gold mine if used well.
So, the first step for organisations to take is to get to know the dark data, and then decide if it is worth keeping or not.