Written by Adrian
Some define Big Data value by use cases. Others describe its advancements by the attributes of Big Data. Ultimately, business capabilities and opportunities result from these Big Data Landscape maturity levels of an enterprise. In this article, the different maturity levels of Big Data and the associated Big Data Science capabilities of enterprises are described.

Picture credits
Enterprise Big Data Maturity
Big Data by different maturity levels has no uniform standard. Some see it as applications levels in the enterprise while others focus more on the capabilities an enterprise has through Big Data.
Commonly, also the application of Big Data plays a role here whereby the focus is less on the data and more on the applications. In different descriptions, the levels of enterprise Big Data capabilities grow step by step.
In the following, we simplify the Big Data landscape maturity of an enterprise to the basic foundations. Thereby, we follow our in-practice learned patterns of how Big Data is often introduced in enterprises.
Level 0: Big Data Landscape planning
In the first state of developing Big Data capabilities, the necessary Big Data and Data Science infrastructure is planned.
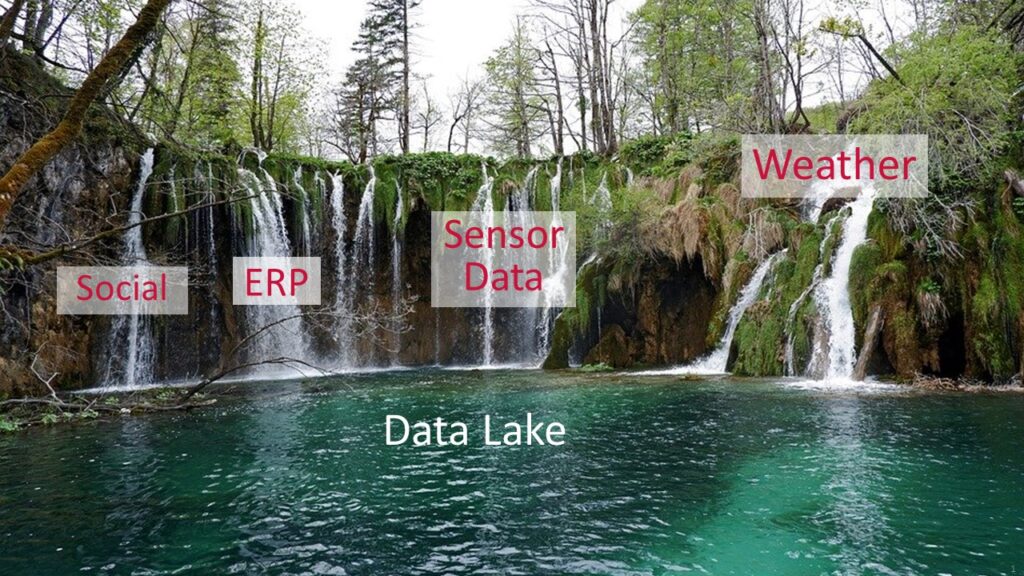
Original picture credits
Data production in various infrastructure systems is happening naturally and maybe the data is even stored in a non-special defined way. The data of the various systems is only consolidated partially in a Data Warehouse.
The first thing that comes to mind is determining which additional data can be stored. This might be data such as Time Series Data in transactional systems which did not get logged before. Maybe IoT gateways to store sensor data are defined or machines are getting equipped with sensors to transmit data in the future.
Use cases are drafted in which Big Data Scenarios can bring value in digitization. First experiments with Big Data tools and exemplary data in a small amount might happen. However, a professional and productive Big Data Landscape and infrastructure are absent, and thus, no real Big Data analysis with enterprise value happens.
Level 1: Simple Big Data Analysis infrastucture
In this Big Data Landscape maturity stage, the first Big Data infrastructure foundation is installed. Often a value prototype to first demonstrate Big Data Science capabilities and provide Big Data value at the same time is manufactured.
Such a Big Data value prototype is often realized in the form of a mixture between the first case exploration and testing with the aspiration to gain the first value out of Data Science.

Picture credits
The Big Data infrastructure gets used for first applications. Different use cases get defined and give the insight that more investment into the Big Data Landscape can generate a potential return.
Typical for this Big Data Landscape maturity is that Data integration happens in most cases manually or without proper monitoring frameworks, generating reliability challenges on the Big Data platform.
Level 2: Big Data Analysis Insights
Big Data Analysis projects are delivering first value. This maturity of the Big Data Landscape leads to the generation of enterprise advancements supported by light data-driven decisions.
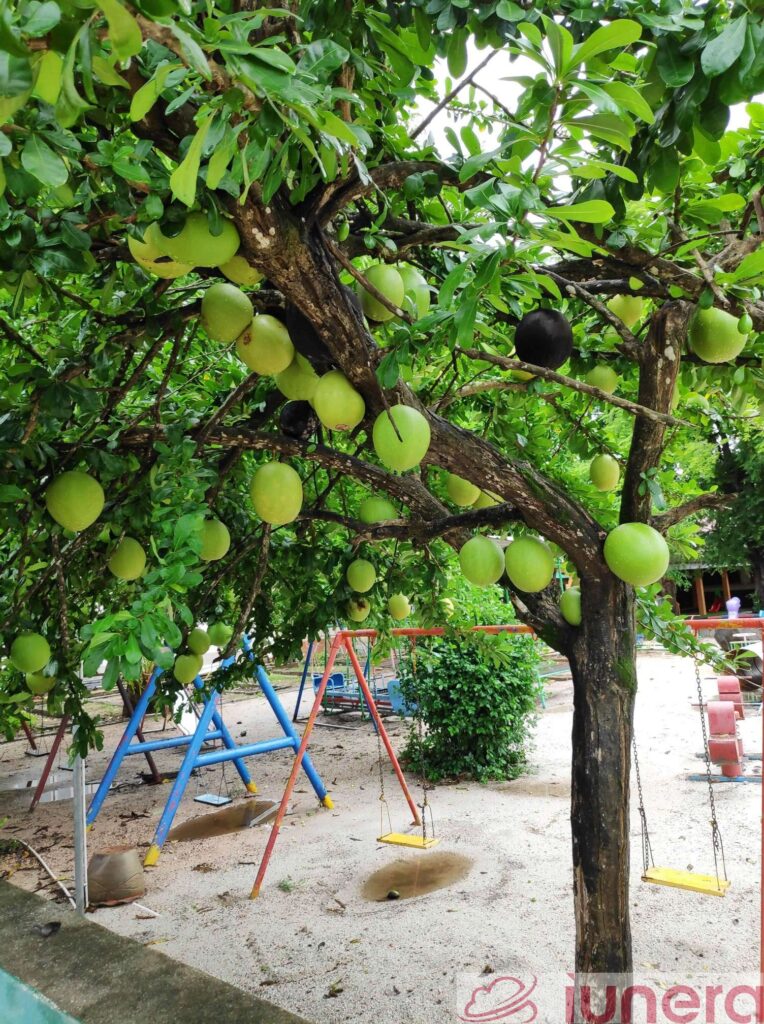
Often the Big Data infrastructure offers some ad-hoc dashboards and more continuous data integrations allow to gain structured overviews for semi-IT-personal when data updates happen.
Typical for this Big Data maturity level is that the first manual applications of machine learning indicate clear AI value. For example, machine learning gets used to doing half-automated classifications which shows new levels of automation that save plenty of manual human labour.
Other first applications allow to gain Big Data analysis insights or to visualize data which was not possible before and gives new insights and overviews. Out of the results, the first use cases to productize the first trial scenarios of this maturity level get identified.
Different challenges like data quality or the necessity for machine learning model management are defined as a to-do. Bottleneck to gain more value out of the Big Data infrastructure is the effort to import data from legacy systems and the lack of use cases that can generate distinctive value at this point in time.
Level 3: Enterprise Big Data Service
Sole Big Data use cases are operationalized and value-added for internal or external customers. The operationalized cases are in the area of machine learning, visualization and complex event processing. Typical for such a service are recommendations, near-real-time reactions of the software, alerting, real-time Big Data dashboarding or automated labeling of document contents by AI.

Picture credits
The Big Data Service is deployed for mostly less crucial areas to identify risks and establish business and technical processes for updates of AI model updates.
The use cases in operation generate new challenges that were not expected in interactions with end users. Processes to handle these situations within the enterprise need to be established. For instance, users might ask chatbots things about suicide and an enterprise needs to decide how they react to such unforeseen situations even if the chatbot was defined and operationalized for other cases.
Level 4: Mastery of Big Data, reactive intelligence, continous progression
In the last step, optimisations of operationalized use cases are executed and more advanced learning techniques get applied.

Picture credits
Thereby, challanges such as model update pipelines and advanced training fine tunings are in focus. Also, more actual research topics become important. Such things can be online learning and applying unsupervised learning techniques to data streams.
The more actual and the more real-time the data is, the more continuous monitoring, investigations and advancements are important.
For example, natural phenomena like virus outbreaks, weekdays or seasons can lead to pattern changes in Time Series data. Such changes in patterns, therefore, need continuous monitoring and improvement in the applied tools and techniques.
Sum-up FAQ
What are the different levels of Big Data Landscape maturity?
What are typical features of first Big Data analysis?
– Data integration happens manually
– The use case is a mixture between Big Data and Data science
– The first use case is supposed to be a prototype to assess the value
– A proper and well maintained Big Data infrastructure is not installed, yet.
What are signs of Big Data Analysis Insights?
– First operative decisions get supported by Big Data Analysis results
– Ad hoc dashboards show insights and value
– Tests of AI show cost saving benefits or potential business speed ups
What is typical for enterprise Big Data services?
– semi-automated alerting and reaction
– real-time Big Data dashboarding
– automated labelling of document contents by AI.
What is happening when the mastery of Big Data Services is reached?
Continuous monitoring of the Big Data and AI Services is still needed to maintain valid results. Also cutting edge research results need to be more and more applied to ensure the best results. Ultimately, continuous improvements are needed to ensure the enterprise stays on top.