I found out about data downtime while watching an online talk about data downtime by Monte Carlo Co-founder and CEO Barr Moses at the Big Data Conference Europe 2020. Watching the talk inspired me to make some Google searches about the topic, so let me share with you what I’ve gathered in this article.
What is data downtime?
The term ‘downtime’ has often been associated with data centres.
“Downtime refers to periods of time during which a computer system, server or network is shut off or unavailable for use.”
The general definition of “downtime” by Webopedia.
This is also applicable to the manufacturing industry, whereby downtime refers to the periods of time in which a company’s factory is not producing products.
There are many different events or issues that can cause downtime, both planned and unplanned, but the downtime that does the most damage to a company is unplanned.
Planned downtime is usually some sort of scheduled maintenance or simply a time when a machine or system isn’t operating because it is not needed at the time.
Meanwhile, unplanned downtime is usually when an unexpected problem shows up during production.
Similarly, Barr defines data downtime as:
“the periods of time when your data is partial, erroneous, missing or otherwise inaccurate.”
In other words, data downtime is the periods of time when data quality is bad or the data is unavailable. You can’t do anything without data or even with bad data.
For example, let’s say you’re forecasting stocks using Twitter as your data source. If Twitter is down, you won’t have any data to use for forecasting. And if Twitter is up and running but has a lot of incorrect data, then your forecasts would be wrong as well.
According to Barr, the symptoms of data downtime can be seen in the following ways:
- Data users are complaining about and slowly losing trust in an organisation’s data.
- An organisation is struggling to adopt data-driven decision making and instead choose a gut-driven approach, which puts the organisation at a disadvantage compared to its competitors.
- The data teams are spending more time on fixing the data downtime than on value-adding activities.
Some common causes of downtime and the costs associated with this business interruption are:
- Human error: accidental deletion or change to data impacts productivity since workers must spend time repeating previous work.
- Ransomware attacks: data can be lost or damaged due to malicious cyber-attacks or through hardware/software faults.
- Hardware failure: can lead to data loss as well.
The cost of data downtime
A 2013 study by Ponemon Institute and Emerson Network Power found that the cost of data centre downtime has been rising.
The average cost per minute of unplanned downtime was US$7,900 in 2013, a staggering 41% increase from US$5,600 per minute in 2010.
The study indicated that downtime has been getting more expensive as data centres started becoming more valuable to their operators.
Many organisations continue to deal with downtime incidents that last from just a few minutes to several days, causing tremendous losses.
Here are some statistics about how much time data teams waste on data downtime, snatching precious time away from activities that drive innovation and generate revenue:
- 50–80% of a data practitioner’s time on collecting, preparing, and fixing “unruly” data.
- 40% of a data analyst’s time on vetting and validating analytics for data quality issues.
- 27% of a salesperson time on dealing with inaccurate data.
- 50% of a data practitioner’s time on identifying, troubleshooting, and fixing data quality, integrity, and reliability issues.
The consequences of leaving data downtime unchecked are similar to what happens when data quality is bad including:
- compliance risk
- lost revenue
- erosion of data trust
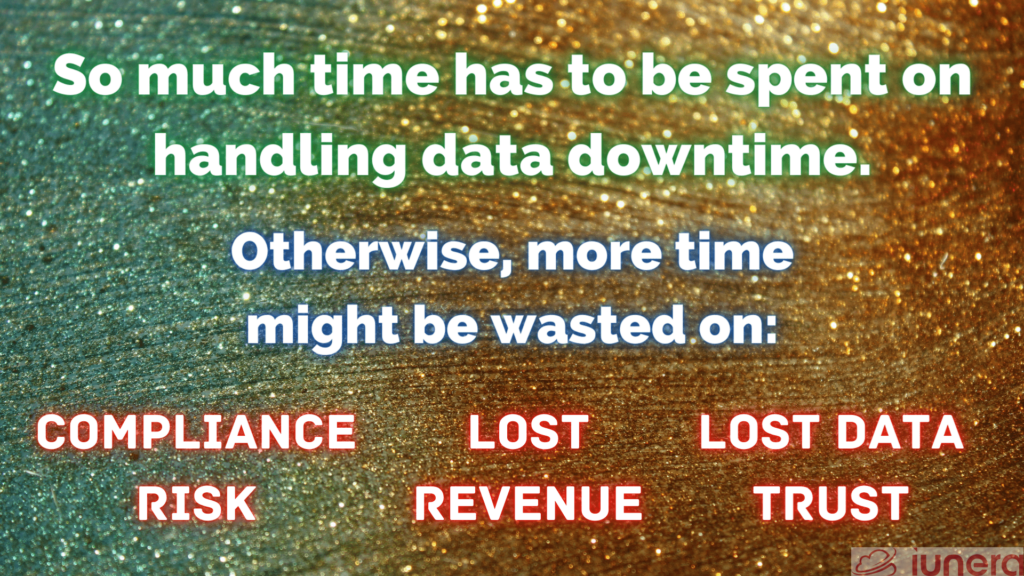
Image designed using Canva.
Examples of data downtime
Major companies such as Amazon and Delta have suffered high profile downtimes before.
Amazon
During the much-anticipated Amazon Prime Day promotion in 2018, some shoppers came across error pages featuring well-known furkids of Amazon employees like this one:
And some other shoppers got stuck in a never-ending loop between the Amazon homepage and a broken “Deals” page.
It was later revealed that the problems were the result of not having enough servers to handle the surge in traffic, which Amazon addressed by manually adding servers, launching a scaled-down version of its homepage and blocking international traffic.
Although Amazon claimed that it was the “biggest shopping event” in company history with over 100 million products sold, the sales number could have been much, much higher without the downtime.
Airline industry
An electrical equipment failure caused the Delta Airlines data centre outage that grounded about 2,000 flights over three days in August 2016.
As a result, Delta had to issue refunds to customers whose flights were cancelled or significantly delayed, costing the airline US$150 million.
Similarly, Southwest Airlines suffered a computer outage in July 2016, which cost at least US$177 million of lost revenue.
In May 2017, a British Airways data centre outage occurred due to human error, causing the cancellation of over 400 flights, which left 75,000 passengers stranded and costed the airline about US$112 million.
Data downtime solutions
There are companies offering downtime solutions like DBTech, Namtek and Thrive.
But just like how a broke young adult will find a daily DIY skincare routine a more affordable option than a professional skincare treatment, organisations could first look for ways to solve data downtime themselves.
The first step in doing this is documenting downtime in reports to help identify the root causes. Reporting downtime can be done by measuring the cost of data downtime.
Monte Carlo has a Data Downtime Cost Calculation equation that considers the resources put into handling data downtime, compliance risk and the opportunity cost of missing revenue-generating activities. Here’s an example:
Labor Cost: ([Number of Engineers] X [Annual Salary of Engineer]) X 30%
+
Compliance Risk: [4% of Your Revenue in 2019]
+
Opportunity Cost: [Revenue you could have generated if you moved faster, releasing X new products, and acquired Y new customers]
= $ Annual Cost of Data Downtime
Upon interviewing and collecting insights from over 80 organisations about their data downtime approach, Barr noticed that, although there isn’t a standard industry best practice, organisations generally follow the data reliability maturity curve.
It’s a journey to maximal data uptime (opposite of data downtime) and has four main steps:
- Reactive: The data team triages a data problem but struggles to use data effectively.
- Proactive: The data team develops manual sanity checks and custom queries to validate their work.
- Automated: The data team has a data health dashboard (like Jira) to view issues, troubleshoot and direct others in the organisation to learn about up-to-date data status.
- Scalable: The data team creates an environment where the team can stay on top of data issues more easily.
As these steps show, it’s much easier to prevent a problem from happening than to cure it once it has happened, so let’s end this article on a proactive note.
Here are some steps that organisations can take to prevent or minimise data downtime such as:
- Monitoring servers and network to ensure that they’re performing optimally.
- Backing up system and data by having three copies of data across two storage media with one backup held offsite for easy recovery in case something goes wrong onsite.
- Keeping up with system upgrades, fixes and cybersecurity best practices.
- Carefully testing and scheduling major upgrades and new systems.
- Training employees to be wary of cybersecurity dangers and to deal with data downtime when it arises.
With all these measures in place, organisations will be in a better position to tackle data downtime before it does any damage.