This post is also available in: Deutsch (German)
The reason why people opt to use public transport is that most of them cannot afford private cars. However, the very same people are the ones who move economies, prompting governments to put out all stops to make sure their transport needs are addressed.
6:00 am New York City: Traffic Congestion
6:00 am Moscow: Traffic Congestion
6:00 am Mumbai: Traffic Congestion
6:00 am Johannesburg: Traffic Congestion
Notice a familiar pattern? Peak hours are this trend’s “synonym”, which happens to be a major headache for public transport operators in populated cities worldwide.
It doesn’t end there. The pattern will repeat in the evening hours as residents emerge from their workplaces in droves and head towards the same direction to board the same Public Service Vehicles (PSVs) that will compete with personal cars for the same resource.
Depending on which city you are from, the result is that a trip that would normally take 45 minutes to complete in off-peak hours takes two hours.
*Word*
To all the people for whom traffic congestion is a lifestyle, a conversation that leads to anything other than a viable solution doesn’t cut it. They have heard that many times before, they are now only interested in a solution to the elephant in the room.
*Bulb glows*
The word that makes the bulb glow in relation to the public transport problems is Big Data.
Below are five reasons why Big Data is the smartest way around public transport challenges.
Occupancy Data
What if there was a way for commuters to know the number of passengers likely to be on a bus or train at a given time even before they board it?
That is not far from reality as occupancy data analysis is now being piloted by some public transport authorities like those of the Australian States of Victoria and New South Wales. There’s a good reason why.
The benefits of analyzing occupancy data are immense including: Knowing the number of passengers aboard a given vehicle or train at a particular time. Currently, this is important because it will help passengers take the lead in enforcing social distancing regulations in public transport.
Besides that, analyzing occupancy data helps predict congestion levels and gives insight into the best routes to use.
The Australian State of Victoria in October 2020 began piloting predictive model tech in its infrastructure to furnish its commuters with real-time data on crowding. The State also began counting passengers in its infrastructure, which was achieved through sensors.
In Sydney, Australia, commuters can check seat availability on trains even before arriving at train stations by looking at the station indicator boards. This is powered by in-built sensors that capture weight data.
That said, there are many other people counting options used by various authorities with the aim of collecting passenger data.
Punctuality
Big Data can also be applied to success to enhance punctuality both for passengers and operators.
The need to apply Big Data by public transport authorities is far much greater in countries like Norway, where harsh weather contributes significantly to late arrivals and departures.
According to a study authored by Ghazal Zakeri and Nils O.E Nelson published in ScienceDirect in 2017 dubbed Investigation of punctuality of local trains – the case of Oslo area, harsh winter and cold weather were the main factors that led to a sharp decline in punctuality between 2007-2010 as per data collected by the operator — Norwegian Railways.
Another study authored by Andrzej Rudnicki published on ScienceDirect states that transport companies must train their efforts on maintaining or reinstating punctuality as it takes precedence in the decision making of a passenger making a trip during specific hours.
How can this be achieved with Big Data?
A thesis paper published by Amsterdam-based Research University Vrije Universiteit proposed smart card & vehicle location data usage to calculate punctuality. The data used for this purpose was provided by GVB, Amsterdam’s public transport company.
The idea was to combine the smart card & vehicle location data sets and establish the punctuality patterns of passengers.
This is one of the ways that Big Data can be leveraged to enhance punctuality.
Demand for Public Transport Routes
Demand for Public Transport Routes is defined as the rate at which most commuters plying a certain route feel road/path x or y is important to them.
If commuters, including those plying different routes, feel that road or path x is critical for their transport needs, the relevant authority will develop better solutions.
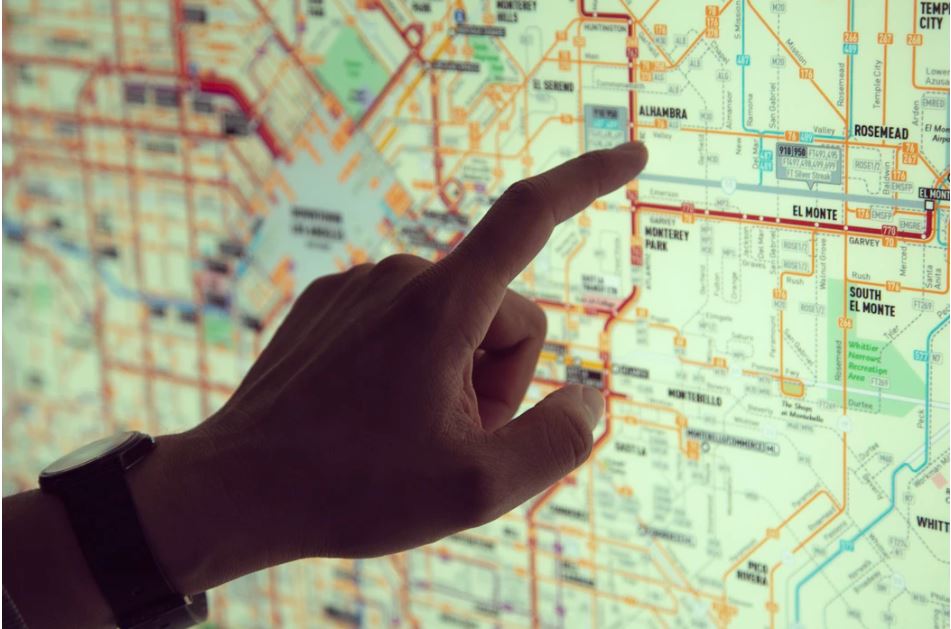
This is best captured in Chapter IV of the Institute of Transportation and Development Policy which states that resources should be spent to benefit the most people instead of political and speculative considerations.
BRT planners generally suggest putting a BRT system in a location that will benefit the most commuters in the best way possible as quickly as possible, most directly through time savings.
Chapter IV of Institute of Transportation and Development Policy
This can be done through the analysis of GTFS data.
Analyzing GTFS data can help inform drivers on the best routes to use, navigate populated cities and towns, and how to reduce the time & cost of traveling.
Multimodal Means of Transport
Walking from home to the train station, taking the train up to the point it alights, taking a bus up to point x and then riding a bicycle to your place of work.
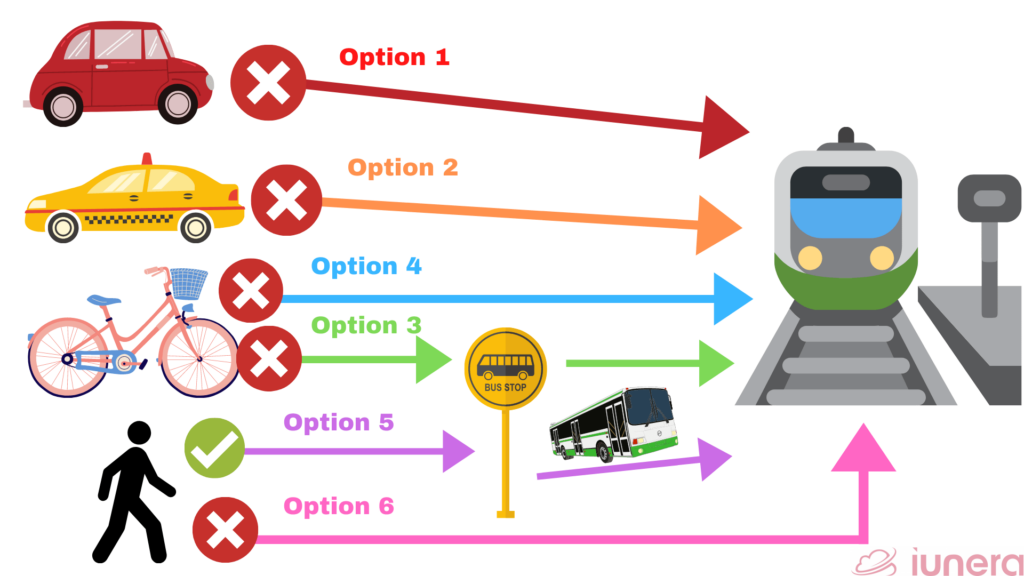
In some countries, this is necessary and the most efficient way to get to where you want to go.
With the advancement of technology, this type of traveling can be optimized further such that a commuter can get to their destination even faster.
Route optimization is defined as establishing the best routes to get to a destination.
How this works is public transport service providers document their routes, after which Big Data experts use the material to forecast the best possible route at a given time.
The next step is for the Big Data experts to work out the best scenarios. The prediction of these routes is done via the route optimization algorithm.
One of the ways Route Optimization was first applied is through the Travel Salesman Problem (TSP).
This was first defined 150 years ago when TSP was racking its brains to figure out exactly how salesmen could reach every one of their customers within the shortest timeframe and using the shortest route available, and return to their destination.
Since then, TSP has evolved and is now widely known as the Vehicle Routing Problem (VRP) and has become even more sophisticated.
Recurring Delays
One question every player in public transport is always trying to answer is how recurring delays can be a thing of the past?
It is that niggling problem that stakeholders cannot seem to shake off.
The common causes of public transport delays include traffic congestion, car breakdowns, bad weather, peak traffic conditions, among other reasons.
According to a research paper published by the University of California Berkeley, recurring delays are a major reason people are likely to give up on public transport.
Well, the answer can be found in Big Data.
One of the ways this can be solved is by analyzing the demand for every route. Once that happens, the most popular routes ought to be earmarked for transport planning and operations. One of the remedies is studying these routes and analyzing patterns and possible causes of delays.
A report authored by Postsavee Prommaharaj, Santi Phithakkitnukoon, Merkebe Getachew Demissie, and Linna Kattan dubbed Visualizing public transit system operation with GTFS data: A case study of Calgary, Canada observed that the use of GTFS Data was reaping dividends in solving problems such as delays.
The research paper sought to assess the impact of a visual analytics tool known as PubtraVis. The tool digests GTFS data that contains schedule information to evaluate and display the public transport system via six visualization modules: density, speed, headway, analysis, flow, and mobility.
The Analysis module displays insightful analytical information including top lists of the most crowded and longest waiting time stations
report: Visualizing public transit system operation with GTFS data: A case study of Calgary, Canada
Commuter is King
If the customer is king in business, then the commuter must be king in public transport.
The reason why people opt to use public transport is that most of them cannot afford private cars. However, the very same people who cannot afford their own cars are the same ones who move economies, so governments put out all stops to make sure their transport needs are addressed.
Big Data is the key to ensuring that happens.
This is espoused by the evolution of the publication of GTFS Data. Authorities publish their transportation data, which is then used by software developers to create applications that solve transport challenges.
Without such developments, there wouldn’t be the next Uber in the pipeline.
But chances are someone is burning the midnight oil somewhere developing the next market-disrupting application.
Thanks to Big Data.